-
Notifications
You must be signed in to change notification settings - Fork 37
/
20-uml.Rmd
583 lines (453 loc) · 18.2 KB
/
20-uml.Rmd
1
2
3
4
5
6
7
8
9
10
11
12
13
14
15
16
17
18
19
20
21
22
23
24
25
26
27
28
29
30
31
32
33
34
35
36
37
38
39
40
41
42
43
44
45
46
47
48
49
50
51
52
53
54
55
56
57
58
59
60
61
62
63
64
65
66
67
68
69
70
71
72
73
74
75
76
77
78
79
80
81
82
83
84
85
86
87
88
89
90
91
92
93
94
95
96
97
98
99
100
101
102
103
104
105
106
107
108
109
110
111
112
113
114
115
116
117
118
119
120
121
122
123
124
125
126
127
128
129
130
131
132
133
134
135
136
137
138
139
140
141
142
143
144
145
146
147
148
149
150
151
152
153
154
155
156
157
158
159
160
161
162
163
164
165
166
167
168
169
170
171
172
173
174
175
176
177
178
179
180
181
182
183
184
185
186
187
188
189
190
191
192
193
194
195
196
197
198
199
200
201
202
203
204
205
206
207
208
209
210
211
212
213
214
215
216
217
218
219
220
221
222
223
224
225
226
227
228
229
230
231
232
233
234
235
236
237
238
239
240
241
242
243
244
245
246
247
248
249
250
251
252
253
254
255
256
257
258
259
260
261
262
263
264
265
266
267
268
269
270
271
272
273
274
275
276
277
278
279
280
281
282
283
284
285
286
287
288
289
290
291
292
293
294
295
296
297
298
299
300
301
302
303
304
305
306
307
308
309
310
311
312
313
314
315
316
317
318
319
320
321
322
323
324
325
326
327
328
329
330
331
332
333
334
335
336
337
338
339
340
341
342
343
344
345
346
347
348
349
350
351
352
353
354
355
356
357
358
359
360
361
362
363
364
365
366
367
368
369
370
371
372
373
374
375
376
377
378
379
380
381
382
383
384
385
386
387
388
389
390
391
392
393
394
395
396
397
398
399
400
401
402
403
404
405
406
407
408
409
410
411
412
413
414
415
416
417
418
419
420
421
422
423
424
425
426
427
428
429
430
431
432
433
434
435
436
437
438
439
440
441
442
443
444
445
446
447
448
449
450
451
452
453
454
455
456
457
458
459
460
461
462
463
464
465
466
467
468
469
470
471
472
473
474
475
476
477
478
479
480
481
482
483
484
485
486
487
488
489
490
491
492
493
494
495
496
497
498
499
500
501
502
503
504
505
506
507
508
509
510
511
512
513
514
515
516
517
518
519
520
521
522
523
524
525
526
527
528
529
530
531
532
533
534
535
536
537
538
539
540
541
542
543
544
545
546
547
548
549
550
551
552
553
554
555
556
557
558
559
560
561
562
563
564
565
566
567
568
569
570
571
572
573
574
575
576
577
578
579
580
581
# Unsupervised Learning
## Introduction
In **unsupervised learning** (UML), no labels are provided, and the
learning algorithm focuses solely on detecting structure in unlabelled
input data. One generally differentiates between
- **Clustering**, where the goal is to find homogeneous subgroups
within the data; the grouping is based on distance between
observations.
- **Dimensionality reduction**, where the goal is to identify patterns in
the features of the data. Dimensionality reduction is often used to
facilitate visualisation of the data, as well as a pre-processing
method before supervised learning.
UML presents specific challenges and benefits:
- there is no single goal in UML
- there is generally much more unlabelled data available than labelled
data.
## k-means clustering
The k-means clustering algorithms aims at partitioning *n*
observations into a fixed number of *k* clusters. The algorithm will
find homogeneous clusters.
In R, we use
```{r, eval=FALSE}
stats::kmeans(x, centers = 3, nstart = 10)
```
where
- `x` is a numeric data matrix
- `centers` is the pre-defined number of clusters
- the k-means algorithm has a random component and can be repeated
`nstart` times to improve the returned model
> Challenge:
>
> - To learn about k-means, let's use the `iris` dataset with the sepal and
> petal length variables only (to facilitate visualisation). Create
> such a data matrix and name it `x`
```{r solirisx, echo=FALSE}
i <- grep("Length", names(iris))
x <- iris[, i]
```
> - Run the k-means algorithm on the newly generated data `x`, save
> the results in a new variable `cl`, and explore its output when
> printed.
```{r solkmcl, echo=FALSE}
cl <- kmeans(x, 3, nstart = 10)
```
> - The actual results of the algorithms, i.e. the cluster membership
> can be accessed in the `clusters` element of the clustering result
> output. Use it to colour the inferred clusters to generate a figure
> like that shown below.
```{r solkmplot, echo=FALSE, fig.cap = "k-means algorithm on sepal and petal lengths"}
plot(x, col = cl$cluster)
```
<details>
```{r soliris, eval=FALSE}
i <- grep("Length", names(iris))
x <- iris[, i]
cl <- kmeans(x, 3, nstart = 10)
plot(x, col = cl$cluster)
```
</details>
### How does k-means work
**Initialisation**: randomly assign class membership
```{r kmworksinit, fig.cap="k-means random intialisation"}
set.seed(12)
init <- sample(3, nrow(x), replace = TRUE)
plot(x, col = init)
```
**Iteration**:
1. Calculate the centre of each subgroup as the average position of
all observations is that subgroup.
2. Each observation is then assigned to the group of its nearest
centre.
It's also possible to stop the algorithm after a certain number of
iterations, or once the centres move less than a certain distance.
```{r kmworksiter, fig.width = 12, fig.cap="k-means iteration: calculate centers (left) and assign new cluster membership (right)"}
par(mfrow = c(1, 2))
plot(x, col = init)
centres <- sapply(1:3, function(i) colMeans(x[init == i, ], ))
centres <- t(centres)
points(centres[, 1], centres[, 2], pch = 19, col = 1:3)
tmp <- dist(rbind(centres, x))
tmp <- as.matrix(tmp)[, 1:3]
ki <- apply(tmp, 1, which.min)
ki <- ki[-(1:3)]
plot(x, col = ki)
points(centres[, 1], centres[, 2], pch = 19, col = 1:3)
```
**Termination**: Repeat iteration until no point changes its cluster
membership.

### Model selection
Due to the random initialisation, one can obtain different clustering
results. When k-means is run multiple times, the best outcome,
i.e. the one that generates the smallest *total within cluster sum of
squares (SS)*, is selected. The total within SS is calculated as:
For each cluster results:
- for each observation, determine the squared euclidean distance from
observation to centre of cluster
- sum all distances
Note that this is a **local minimum**; there is no guarantee to obtain
a global minimum.
> Challenge:
>
> Repeat k-means on our `x` data multiple times, setting the number of
> iterations to 1 or greater and check whether you repeatedly obtain
> the same results. Try the same with random data of identical
> dimensions.
<details>
```{r selrep, fig.width = 12, fig.cap = "Different k-means results on the same (random) data"}
cl1 <- kmeans(x, centers = 3, nstart = 10)
cl2 <- kmeans(x, centers = 3, nstart = 10)
table(cl1$cluster, cl2$cluster)
cl1 <- kmeans(x, centers = 3, nstart = 1)
cl2 <- kmeans(x, centers = 3, nstart = 1)
table(cl1$cluster, cl2$cluster)
set.seed(42)
xr <- matrix(rnorm(prod(dim(x))), ncol = ncol(x))
cl1 <- kmeans(xr, centers = 3, nstart = 1)
cl2 <- kmeans(xr, centers = 3, nstart = 1)
table(cl1$cluster, cl2$cluster)
diffres <- cl1$cluster != cl2$cluster
par(mfrow = c(1, 2))
plot(xr, col = cl1$cluster, pch = ifelse(diffres, 19, 1))
plot(xr, col = cl2$cluster, pch = ifelse(diffres, 19, 1))
```
</details>
### How to determine the number of clusters
1. Run k-means with `k=1`, `k=2`, ..., `k=n`
2. Record total within SS for each value of k.
3. Choose k at the *elbow* position, as illustrated below.
```{r kmelbow, echo=FALSE, fig.cap = ""}
ks <- 1:5
tot_within_ss <- sapply(ks, function(k) {
cl <- kmeans(x, k, nstart = 10)
cl$tot.withinss
})
plot(ks, tot_within_ss, type = "b",
ylab = "Total within squared distances",
xlab = "Values of k tested")
```
> Challenge
>
> Calculate the total within sum of squares for k from 1 to 5 for our
> `x` test data, and reproduce the figure above.
<details>
```{r solkmelbow}
ks <- 1:5
tot_within_ss <- sapply(ks, function(k) {
cl <- kmeans(x, k, nstart = 10)
cl$tot.withinss
})
plot(ks, tot_within_ss, type = "b")
```
</details>
## Hierarchical clustering
### How does hierarchical clustering work
**Initialisation**: Starts by assigning each of the n points its own cluster
**Iteration**
1. Find the two nearest clusters, and join them together, leading to
n-1 clusters
2. Continue the cluster merging process until all are grouped into a
single cluster
**Termination:** All observations are grouped within a single cluster.
```{r hcldata, fig.width = 12, echo=FALSE, fig.cap = "Hierarchical clustering: initialisation (left) and colour-coded results after iteration (right)."}
set.seed(42)
xr <- data.frame(x = rnorm(5),
y = rnorm(5))
cls <- c("red", "blue", "orange", "blue", "orange")
cls <- scales::col2hcl(cls, alpha = 0.5)
par(mfrow = c(1, 2))
plot(xr, cex = 3)
text(xr$x, xr$y, 1:5)
plot(xr, cex = 3, col = cls, pch = 19)
text(xr$x, xr$y, 1:5)
```
The results of hierarchical clustering are typically visualised along
a **dendrogram**, where the distance between the clusters is
proportional to the branch lengths.
```{r hcldendro, echo=FALSE, fig.cap = "Visualisation of the hierarchical clustering results on a dendrogram"}
plot(hcr <- hclust(dist(xr)))
```
In R:
- Calculate the distance using `dist`, typically the Euclidean
distance.
- Hierarchical clustering on this distance matrix using `hclust`
> Challenge
>
> Apply hierarchical clustering on the `iris` data and generate a
> dendrogram using the dedicated `plot` method.
<details>
```{r hclsol, fig.cap = ""}
d <- dist(iris[, 1:4])
hcl <- hclust(d)
hcl
plot(hcl)
```
</details>
### Defining clusters
After producing the hierarchical clustering result, we need to *cut
the tree (dendrogram)* at a specific height to defined the
clusters. For example, on our test dataset above, we could decide to
cut it at a distance around 1.5, with would produce 2 clusters.
```{r cuthcl, echo=FALSE, fig.cap = "Cutting the dendrogram at height 1.5."}
plot(hcr)
abline(h = 1.5, col = "red")
```
In R we can us the `cutree` function to
- cut the tree at a specific height: `cutree(hcl, h = 1.5)`
- cut the tree to get a certain number of clusters: `cutree(hcl, k = 2)`
> Challenge
>
> - Cut the iris hierarchical clustering result at a height to obtain
> 3 clusters by setting `h`.
> - Cut the iris hierarchical clustering result at a height to obtain
> 3 clusters by setting directly `k`, and verify that both provide
> the same results.
<details>
```{r cuthclsol}
plot(hcl)
abline(h = 3.9, col = "red")
cutree(hcl, k = 3)
cutree(hcl, h = 3.9)
identical(cutree(hcl, k = 3), cutree(hcl, h = 3.9))
```
</details>
> Challenge
>
> Using the same value `k = 3`, verify if k-means and hierarchical
> clustering produce the same results on the `iris` data.
>
> Which one, if any, is correct?
<details>
```{r iris2algs, fig.width = 12, fig.cap = ""}
km <- kmeans(iris[, 1:4], centers = 3, nstart = 10)
hcl <- hclust(dist(iris[, 1:4]))
table(km$cluster, cutree(hcl, k = 3))
par(mfrow = c(1, 2))
plot(iris$Petal.Length, iris$Sepal.Length, col = km$cluster, main = "k-means")
plot(iris$Petal.Length, iris$Sepal.Length, col = cutree(hcl, k = 3), main = "Hierarchical clustering")
## Checking with the labels provided with the iris data
table(iris$Species, km$cluster)
table(iris$Species, cutree(hcl, k = 3))
```
</details>
## Pre-processing
Many of the machine learning methods that are regularly used are
sensitive to difference scales. This applies to unsupervised methods
as well as supervised methods, as we will see in the next chapter.
A typical way to pre-process the data prior to learning is to scale
the data, or apply principal component analysis (next section). Scaling
assures that all data columns have a mean of 0 and standard deviation of 1.
In R, scaling is done with the `scale` function.
> Challenge
>
> Using the `mtcars` data as an example, verify that the variables are
> of different scales, then scale the data. To observe the effect
> different scales, compare the hierarchical clusters obtained on the
> original and scaled data.
<details>
```{r scalesol, fig.width=12, fig.cap=""}
colMeans(mtcars)
hcl1 <- hclust(dist(mtcars))
hcl2 <- hclust(dist(scale(mtcars)))
par(mfrow = c(1, 2))
plot(hcl1, main = "original data")
plot(hcl2, main = "scaled data")
```
</details>
## Principal component analysis (PCA)
**Dimensionality reduction** techniques are widely used and versatile
techniques that can be used to:
- find structure in features
- pre-processing for other ML algorithms, and
- aid in visualisation.
The basic principle of dimensionality reduction techniques is to
transform the data into a new space that summarise properties of the
whole data set along a reduced number of dimensions. These are then
ideal candidates used to visualise the data along these reduced number
of informative dimensions.
### How does it work
Principal Component Analysis (PCA) is a technique that transforms the
original n-dimensional data into a new n-dimensional space.
- These new dimensions are linear combinations of the original data,
i.e. they are composed of proportions of the original variables.
- Along these new dimensions, called principal components, the data
expresses most of its variability along the first PC, then second,
...
- Principal components are orthogonal to each other,
i.e. non-correlated.
```{r pcaex, echo=FALSE, fig.width=12, fig.height=4, fig.cap="Original data (left). PC1 will maximise the variability while minimising the residuals (centre). PC2 is orthogonal to PC1 (right)."}
set.seed(1)
xy <- data.frame(x = (x <- rnorm(50, 2, 1)),
y = x + rnorm(50, 1, 0.5))
pca <- prcomp(xy)
z <- cbind(x = c(-1, 1), y = c(0, 0))
zhat <- z %*% t(pca$rotation[, 1:2])
zhat <- scale(zhat, center = colMeans(xy), scale = FALSE)
par(mfrow = c(1, 3))
plot(xy, main = "Orignal data (2 dimensions)")
plot(xy, main = "Orignal data with PC1")
abline(lm(y ~ x, data = data.frame(zhat - 10)), lty = "dashed")
grid()
plot(pca$x, main = "Data in PCA space")
grid()
```
In R, we can use the `prcomp` function.
Let's explore PCA on the `iris` data. While it contains only 4
variables, is already becomes difficult to visualise the 3 groups
along all these dimensions.
```{r irispairs, fig.cap=""}
pairs(iris[, -5], col = iris[, 5], pch = 19)
```
Let's use PCA to reduce the dimension.
```{r irispca}
irispca <- prcomp(iris[, -5])
summary(irispca)
```
A summary of the `prcomp` output shows that along PC1 along, we are
able to retain over 92% of the total variability in the data.
```{r histpc1, echo=FALSE, fig.cap="Iris data along PC1."}
## boxplot(irispca$x[, 1] ~ iris[, 5], ylab = "PC1")
hist(irispca$x[iris$Species == "setosa", 1],
xlim = range(irispca$x[, 1]), col = "#FF000030",
xlab = "PC1", main = "PC1 variance explained 92%")
rug(irispca$x[iris$Species == "setosa", 1], col = "red")
hist(irispca$x[iris$Species == "versicolor", 1], add = TRUE, col = "#00FF0030")
rug(irispca$x[iris$Species == "versicolor", 1], col = "green")
hist(irispca$x[iris$Species == "virginica", 1], add = TRUE, col = "#0000FF30")
rug(irispca$x[iris$Species == "virginica", 1], col = "blue")
```
### Visualisation
A **biplot** features all original points re-mapped (rotated) along the
first two PCs as well as the original features as vectors along the
same PCs. Feature vectors that are in the same direction in PC space
are also correlated in the original data space.
```{r irisbiplot, fig.cap=""}
biplot(irispca)
```
One important piece of information when using PCA is the proportion of
variance explained along the PCs, in particular when dealing with high
dimensional data, as PC1 and PC2 (that are generally used for
visualisation), might only account for an insufficient proportion of
variance to be relevant on their own.
In the code chunk below, I extract the standard deviations from the
PCA result to calculate the variances, then obtain the percentage of
and cumulative variance along the PCs.
```{r irispcavar}
var <- irispca$sdev^2
(pve <- var/sum(var))
cumsum(pve)
```
> Challenge
>
> - Repeat the PCA analysis on the iris dataset above, reproducing the
> biplot and preparing a barplot of the percentage of variance
> explained by each PC.
> - It is often useful to produce custom figures using the data
> coordinates in PCA space, which can be accessed as `x` in the
> `prcomp` object. Reproduce the PCA plots below, along PC1 and PC2
> and PC3 and PC4 respectively.
```{r irispcax, echo=FALSE, fig.width=12, fig.cap=""}
par(mfrow = c(1, 2))
plot(irispca$x[, 1:2], col = iris$Species)
plot(irispca$x[, 3:4], col = iris$Species)
```
<details>
```{r irispcaxcol, eval=FALSE}
par(mfrow = c(1, 2))
plot(irispca$x[, 1:2], col = iris$Species)
plot(irispca$x[, 3:4], col = iris$Species)
```
</details>
### Data pre-processing
We haven't looked at other `prcomp` parameters, other that the first
one, `x`. There are two other ones that are or importance, in
particular in the light of the section on pre-processing above, which
are `center` and `scale.`. The former is set to `TRUE` by default,
while the second one is set the `FALSE`.
> Challenge
>
> Repeat the analysis comparing the need for scaling on the `mtcars`
> dataset, but using PCA instead of hierarchical clustering. When
> comparing the two.
<details>
```{r scalepcasol, fig.with=12, fig.cap=""}
par(mfrow = c(1, 2))
biplot(prcomp(mtcars, scale = FALSE), main = "No scaling") ## 1
biplot(prcomp(mtcars, scale = TRUE), main = "With scaling") ## 2
```
Without scaling, `disp` and `hp` are the features with the highest
loadings along PC1 and 2 (all others are negligible), which are also
those with the highest units of measurement. Scaling removes this
effect. </details>
### Final comments on PCA
Real datasets often come with **missing values**. In R, these should
be encoded using `NA`. Unfortunately, PCA cannot deal with missing
values, and observations containing `NA` values will be dropped
automatically. This is a viable solution only when the proportion of
missing values is low.
It is also possible to impute missing values. This is described in
greater details in the *Data pre-processing* section in the supervised
machine learning chapter.
Finally, we should be careful when using categorical data in any of
the unsupervised methods described above. Categories are generally
represented as factors, which are encoded as integer levels, and might
give the impression that a distance between levels is a relevant
measure (which it is not, unless the factors are ordered). In such
situations, categorical data can be dropped, or it is possible to
encode categories as binary **dummy variables**. For example, if we
have 3 categories, say `A`, `B` and `C`, we would create two dummy
variables to encode the categories as:
```{r dummvar, echo=FALSE}
dfr <- data.frame(x = c(1, 0, 0),
y = c(0, 1, 0))
rownames(dfr) <- LETTERS[1:3]
knitr::kable(dfr)
```
so that the distance between each category are approximately equal to
1.
## t-Distributed Stochastic Neighbour Embedding
[t-Distributed Stochastic Neighbour Embedding](https://lvdmaaten.github.io/tsne/) (t-SNE)
is a *non-linear* dimensionality reduction technique, i.e. that
different regions of the data space will be subjected to different
transformations. t-SNE will compress small distances, thus bringing
close neighbours together, and will ignore large distances. It is
particularly well suited
for
[very high dimensional data](https://distill.pub/2016/misread-tsne/).
In R, we can use the `Rtsne` function from the `r CRANpkg("Rtsne")`.
Before, we however need to remove any duplicated entries in the
dataset.
```{r iristsne, fig.cap=""}
library("Rtsne")
uiris <- unique(iris[, 1:5])
iristsne <- Rtsne(uiris[, 1:4])
plot(iristsne$Y, col = uiris$Species)
```
As with PCA, the data can be scaled and centred prior the running
t-SNE (see the `pca_center` and `pca_scale` arguments). The algorithm
is stochastic, and will produce different results at each repetition.
### Parameter tuning
t-SNE (as well as many other methods, in particular classification
algorithms) has two important parameters that can substantially
influence the clustering of the data
- **Perplexity**: balances global and local aspects of the data.
- **Iterations**: number of iterations before the clustering is
stopped.
It is important to adapt these for different data. The figure below
shows a 5032 by 20 dataset that represent protein sub-cellular
localisation.
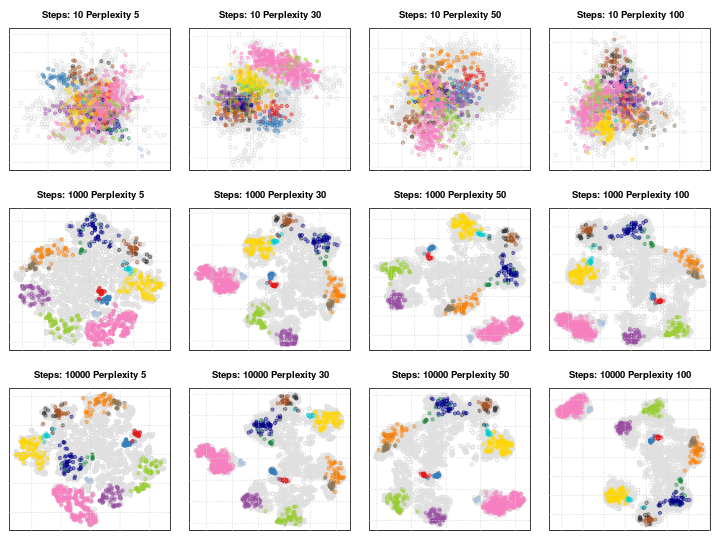
As a comparison, below are the same data with PCA (left) and t-SNE
(right).
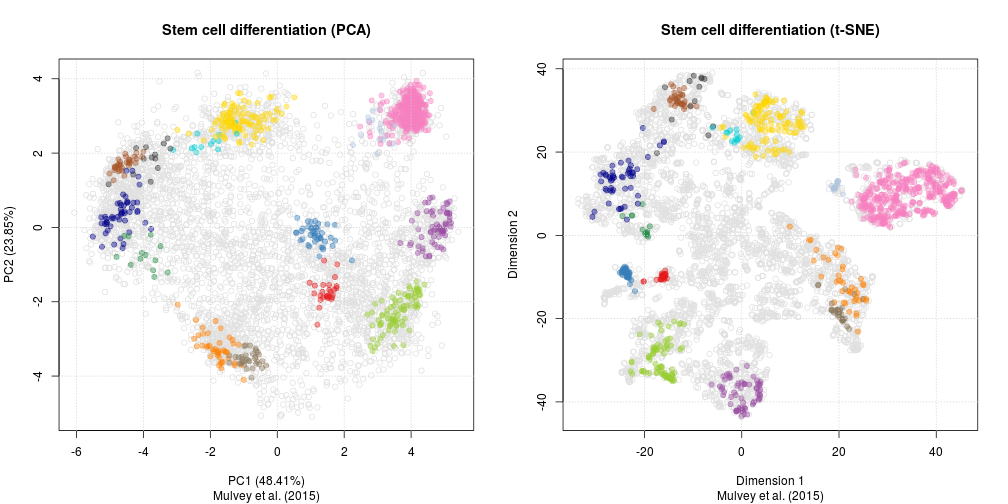